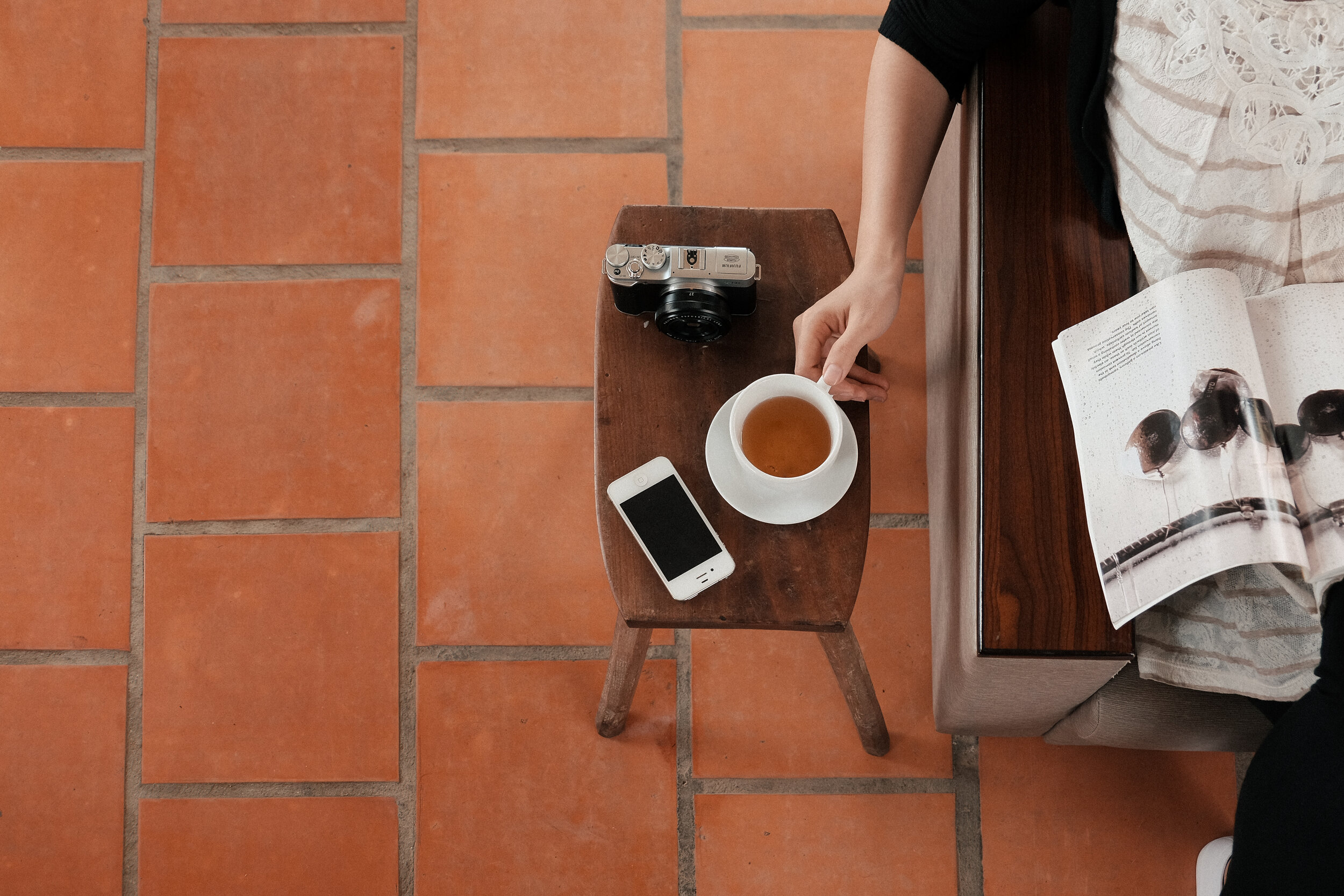
Media giant enhances content and finds new markets with text analytics
The Tribune Content Agency (formerly McClatchy-Tribune Information Services), provides content to media outlets, content aggregators and online databases. Bacon Tree Consulting principals helped TCA create a product called TCA SmartContent, which provides small, relevant subsets of content to media outlets and the electronic marketplace.
TCA's goals were to:
grow revenue,
improve internal search,
protect business by adding value for traditional clients, and
create value for its media base through enhanced search and mobile apps.
Regional News, one of TCA's divisions, brings in and redistributes 2 million news articles and blog posts a year. TCA was tagging this content in two ways: editors curated articles and added traditional newspaper tags called "slugs" to them, and an automated process gathered content from media sites and minimally processed them before delivering them to clients.
Fig. 1, is an example of the system that TCA used to tag articles as they were distributed across the news wire. The first item in the list is 50 Plus, also referred to as Baby Boomers. Editors selected articles to tag with the 50 Plus label and were able to select from a broad range of topics that appeal to Baby Boomers, including travel, fitness, fashion and health insurance. That diversity is a good example of how manual tagging can force content into different categories.
However, about 90 percent of TCA's content was processed through the automated system, which had even broader tagging denoting the traditional sections of a newspaper, such as local, sports, business and national.
TCA needed to bring these two systems into better alignment and, as importantly, to provide clients with a way to find the precise content they needed within the 5,500 articles they processed a day.
Add, classify, test, repeat
TCA previously had a firm auto-classifying its content, but that project had never gotten above a 25 percent accuracy rate in the four years it was in place. One of the contributing factors was poorly communicated business and technical needs at the beginning of the project. This pre-disposed TCA's leaders to bring the project in-house, where they would have more control over the process of classification.
After interviewing stakeholders, we decided to build a taxonomy for news that TCA could use in auto-classification and to drive search on its website.
We first focused on building solid information architecture, which meant we needed to make sure that the:
business stakeholders understood the needs of the customers,
business needs were translated into technical requirements,
management was up to date and on board, and
we had set an achievable time line.
We created a cross-functional team of editors, business owners, subject matter experts, and management to make sure all needs were represented in goals and design of the project. This exercise was essential to building a taxonomy that fit TCA's early needs and to create a roadmap to ongoing development.
We started by adding traditional newspaper sections to the taxonomy structure. We then added IPTC (International Press Telecommunications Council) codes and mapped those to the traditional newspaper sections and Associated Press codes. We loaded those into a taxonomy management tool, and started developing more depth to suit TCA's content.
We decided to use editors to build the taxonomy because editors know news content better than technologists do. Specifically, editors:
know words and how they work together,
understand how reporters write,
know what the structure of a news story is, and
see how news subject relate to one another.
We set up a system where editors added a broad taxonomy of terms and synonyms, created rule-bases with those terms and classified content. We then tested how those terms performed in auto-classification against a day's worth of content. These broad concepts helped catch similar content that we then looked at for more specific themes. Those themes became the next, more narrow set of concepts. We repeated the process for each tier until we had developed the specificity we needed. To sum up, we added, classified, tested, repeated.
Once a document was classified, TCA's custom content management system stored XML with the classification of each piece of content. Each story gets a set of tags that might denote topics, geography, dates, currencies, companies, the function of the document, and other data points. Searching on those returns highly relevant results. It is the difference between finding the last five stories written on exactly the topic you’re looking for and having to sift through a million returns from an Internet search. This ability to get precise, relevant search results is the power of TCA SmartContent.
Auto-classification increases revenue for TCA
As a result, TCA went to market with its niche news product, TCA SmartContent, three months after we started building their ontology. Users of the TCA SmartContent site are able to create feeds by browsing through the ontology. They can save feeds for later use and can have content sent to them as frequently as they'd like.
Now, media clients receive the subset of articles they need without having to do a complicated series of searches through more than 5,000 articles a day. Online databases receive metadata along with news articles that they can use to help their clients search current and archived content. Other clients create newsletters with a content set that is small, but precise enough to curate by hand.
The ontology gives TCA the ability to automatically deliver content to websites, push content on specific subjects to mobile users based on user profiles, tailor the reading experience by showing content on web pages that they already know are of interest to individual readers, and serve custom content based on advertisers' products. Related tags can be used to power internal search engines on customers' sites and as an internal library for research on whole stories or parts of news items.
In its second year, TCA saw a 400 percent revenue growth over the year before – and all of it without adding staff. The time they spent on taxonomy development they gained on automating other tasks through metadata use.
TCA continues to build its taxonomy with the help of BTC principals. Using this increasingly-well developed taxonomy, they are able to classify more esoteric concepts, such as the Baby Boomers topic that editors were applying manually at the beginning of the project, that cover a broad set of topics.